In the dynamic landscape of Language Models (LLMs), a new buzzword is making waves – RAG, short for Retrieval-Augmented Generation. This innovative approach transforms the capabilities of LLMs by seamlessly integrating external information into the language generation process. In this blog, we explore the intricacies of RAG and how it opens up a realm of possibilities for enterprises looking to enhance their responses and create a more human-like conversational interface.

Understanding RAG: Retrieval Augmented Generation
RAG fundamentally operates by marrying the strengths of neural language models with the wealth of information available in external textual data. This synergy is particularly advantageous for enterprises seeking to bolster their responses using LLMs like GPT-4, Claude, and Llama.
Components of RAG
- Retriever: This element is responsible for locating relevant documents or passages in response to a query. Leveraging similarity search algorithms, the retriever sifts through extensive datasets to pinpoint the most pertinent information.
- Generator: Once the retriever acquires the relevant information, the generator utilizes it to construct a response. This component, a language model, considers both the initial query and the retrieved documents to generate coherent and contextually relevant answers.
Realizing the Potential of RAG in Enterprise Applications
- Product Information: RAG ensures accurate and up-to-date details about product features by retrieving the latest specifications or manuals from the company’s database.
- Order Tracking: The system excels at providing real-time updates on order status by retrieving information from the shipping partner’s API or database.
- Handling Returns: RAG retrieves the most current return policies, guiding customers through the process with precision.
- Troubleshooting: For technical support, the chatbot uses RAG to access troubleshooting guides or the latest technical bulletins, facilitating swift issue resolution.
Implementing RAG with Confluence Knowledge Base
To leverage RAG with a knowledge base stored in Confluence, a robust system is required. The retriever accesses the Confluence API to search and index relevant documents, forming the foundation for the RAG model. This integration enhances information retrieval tasks within the enterprise, offering immense value for customer support, internal knowledge sharing, and various applications requiring specific information from the company’s knowledge base.
Conclusion: Empowering Enterprises with RAG
In the era of advanced language models, RAG emerges as a powerful tool for enterprises. By combining the capabilities of LLMs with real-time external data, enterprises can provide responses that are not only contextually relevant but also based on the most current and specific information available. As we embrace the era of RAG, enterprises can unlock the full potential of language models, ensuring efficient and customer-centric communication.
About Author
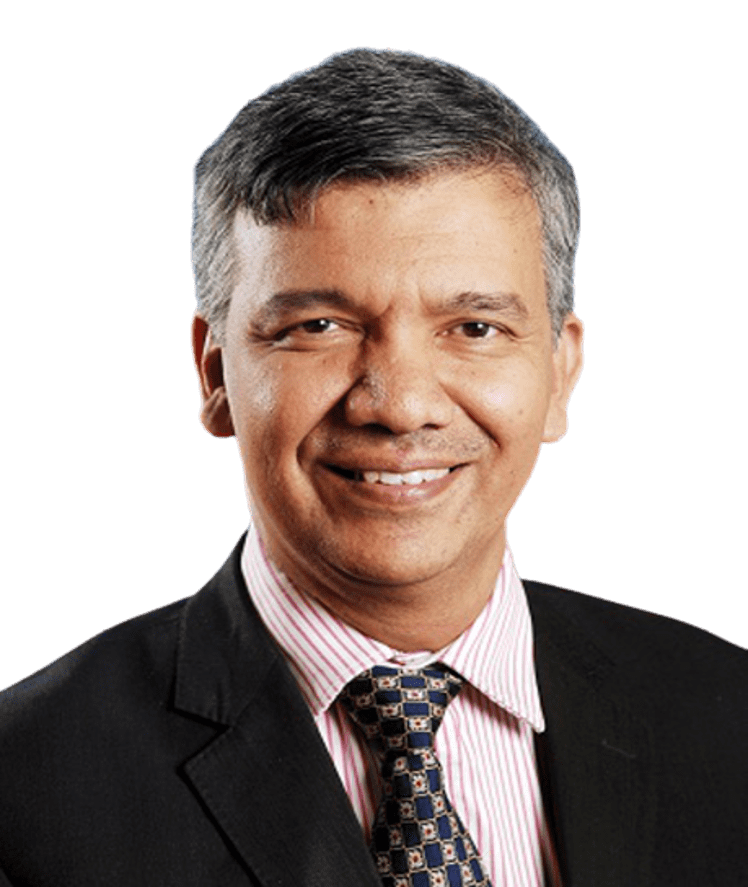
Ram is a Cloud Security Expert with 30+ years of IT experience, holding 26 patents in Infra, AI-ML, and Automation. He’s a Wipro Fellow, an Independent Consultant for Fortune 15 companies, and has won international awards for Automation. Ram’s cost rationalization work benefited enterprises like Citi Bank, Credit Suisse, and UBS.